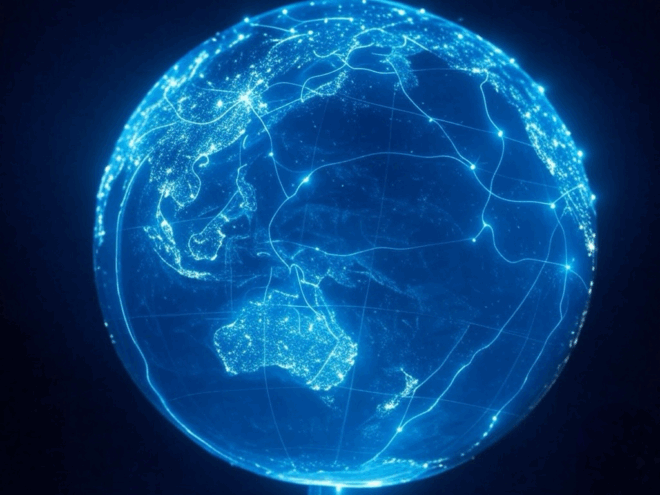
Introduction
Human civilisation is an ongoing experiment, characterised by continuous adaptation to technological, economic, and social changes. Political systems, often presented as enduring solutions, are inherently transient, shaped by the tools, resources, and material conditions of their era. From the agrarian monarchies of antiquity to the industrial democracies of the modern age, governance has evolved to harness new realities and address emerging challenges. Democratic capitalism, fuelled by the steam-powered Industrial Revolution, was once heralded as the pinnacle of modernity, promising both individual liberty and collective prosperity. However, it now falters in the Information Age and faces existential challenges in the impending AI revolution.
This exploration synthesises three critical dimensions: the impermanence of political systems, potential governance models for an AI-driven world, and ethical frameworks to ensure responsible AI development. It argues that conservative resistance to change – through nostalgia for industrial-era systems or earlier paradigms – is not only futile but dangerous, risking societal stagnation amid transformative technological shifts. Instead, societies must embrace experimentation, drawing on historical lessons and ethical principles to design governance systems that align with AI’s potential and mitigate its risks. The conclusion advocates for adaptive, inclusive, and forward-looking approaches to navigate the AI revolution, ensuring it fosters equity, accountability, and human flourishing.
The Technological Foundations of Governance
Governance systems are not abstract ideals but pragmatic responses to the technological and economic realities of their time. Technologies – whether the plow, the steam engine, or the internet – shape power dynamics, social organisation, and resource allocation, which in turn define the contours of political structures.
1. Agrarian Societies and Hierarchical Governance
In pre-industrial societies, agriculture was the backbone of economic and social life, demanding centralised control over land, labor, and resources. Monarchies, feudal systems, and tribal hierarchies emerged as efficient mechanisms for managing scarce resources, enforcing order, and mobilising populations for collective tasks like irrigation or defence. The slow pace of technological change – limited to incremental improvements in tools and farming techniques – reinforced the stability of these systems, which persisted for centuries. Governance was rigid, hierarchical, and localised, reflecting the constraints of manual labor and geographically bound economies.
2. The Industrial Revolution and Democratic Capitalism
The advent of the steam engine and mechanised production in the 18th and 19th centuries disrupted agrarian hierarchies, ushering in the Industrial Revolution. Factories, urban centers, and global trade created new economic realities, including a burgeoning middle class and unprecedented wealth generation. Democratic capitalism arose as a response, balancing individual liberty with collective governance to harness industrial innovation. The United States, with its constitutional framework emphasising checks and balances, became a beacon of this model, blending democratic participation with market-driven prosperity. By the mid-20th century, democratic capitalism was widely regarded as the apex of political organisation, promising freedom, opportunity, and progress. Its success was tied to industrial technologies that enabled mass production, global trade, and social mobility.
3. The Information Age and the Cracks in the System
The rise of digital technologies – computers, the internet, and global communication networks – ushered in the Information Age, exposing the limitations of democratic capitalism. The rapid dissemination of information empowered individuals, enabling grassroots movements and global connectivity. However, it also amplified polarisation, misinformation, and economic inequality. Globalised markets eroded national sovereignty, as multinational corporations and tech giants wielded power that traditional democratic institutions struggled to regulate. Social media platforms, for instance, shaped public discourse in ways that electoral systems could not control, while data-driven economies concentrated wealth in the hands of a few. Designed for a slower, industrial world, democratic capitalism began to falter under the pressures of instantaneous data flows, borderless economies, and algorithmic influence.
The AI Revolution: A New Paradigm
The AI revolution, already underway, promises to fundamentally reshape civilisation. Artificial intelligence – capable of autonomous decision-making, vast data processing, and rapid scalability – will redefine labor, wealth, power, and social structures. Democratic capitalism, tethered to industrial and informational paradigms, is ill-equipped to navigate this transformation, necessitating new governance models and ethical frameworks.
1. Economic Disruption
AI-driven automation threatens to displace entire sectors of the workforce, from manufacturing to white-collar professions like law and medicine. The International Labour Organisation estimates that up to 30% of current jobs could be automated by 2030, exacerbating inequality and rendering traditional labor-based economies obsolete. The capitalist model, which relies on wage labor and consumer markets, faces collapse if vast populations are rendered economically redundant. Concepts like universal basic income (UBI) are gaining traction as potential stopgaps, but they challenge the core assumptions of market-driven systems. For instance, UBI trials in Finland and Canada have shown mixed results, highlighting the need for broader systemic reforms to address AI-driven economic shifts.
2. Governance Challenges
AI’s ability to process massive datasets and make autonomous decisions raises profound questions about accountability, control, and legitimacy. Democratic institutions, designed for human deliberation and periodic elections, struggle to regulate algorithms that operate at superhuman speed and complexity. For example, AI-driven financial trading systems can execute millions of transactions per second, outpacing regulatory oversight. Moreover, the concentration of AI capabilities in the hands of a few corporations (e.g., Google, Amazon) or states (e.g., China, US) risks creating new forms of technocratic authoritarianism, where power is wielded by those who control the algorithms. This undermines democratic ideals of transparency and public accountability.
3. Social Transformation
AI will reshape social structures, from education to community organisation. Generative AI tools, such as large language models, are transforming how knowledge is created and shared, while virtual and augmented realities blur the lines between physical and digital worlds. These shifts may erode traditional notions of citizenship, civic engagement, and community, as individuals increasingly interact in decentralised, algorithm-mediated spaces. Governance systems must adapt to these fluid realities, potentially favouring networked, participatory models over rigid, state-centric hierarchies. For instance, online platforms like Reddit or decentralised autonomous organisations (DAOs) hint at new forms of collective decision-making that could replace traditional governance structures.
The Futility of Conservative Resistance
Conservative impulses to “turn back time” and preserve democratic capitalism – or revert to earlier systems like agrarian hierarchies – ignore the inexorable link between technology and governance. Nostalgia for a perceived golden age, whether the industrial prosperity of the 1950s or the cultural homogeneity of earlier eras, disregards the material conditions that made those systems viable.
1. The Myth of Restoration
Efforts to restore industrial-era governance – through protectionist trade policies, deregulation, or cultural conservatism – fail to address the realities of a post-industrial, AI-driven world. For example, policies aimed at reviving manufacturing jobs, such as tariffs on imported goods, cannot compete with AI-driven automation, which reduces labor costs to near zero. Similarly, attempts to curb digital platforms through censorship or antitrust measures overlook their integral role in the modern economy, from e-commerce to social connectivity. These efforts are akin to trying to revive horse-drawn carriages in the age of automobiles – well-intentioned but ultimately futile.
2. The Danger of Stagnation
Clinging to outdated systems risks societal stagnation and collapse. History is replete with examples of civilisations that failed to adapt to technological change. The Roman Empire’s inability to manage its sprawling infrastructure led to its decline, while the Qing Dynasty’s resistance to industrialisation left China vulnerable to colonial powers. Democratic capitalism, if preserved beyond its technological relevance, could similarly implode under the pressures of AI-driven disruption, as economic inequality, social unrest, and ungoverned technologies destabilise societies.
3. The Need for Forward-Looking Adaptation
Rather than resisting change, societies must embrace experimentation and innovation. Governance in the AI era may require hybrid systems that blend democratic participation with technocratic expertise, or entirely new models that prioritise resilience and equity in a post-scarcity world. The specifics remain uncertain, but historical transitions – from feudalism to democracy – suggest that flexibility, inclusivity, and a willingness to iterate are critical to success.
AI Governance Models
To address the challenges and opportunities of the AI revolution, governance models must balance innovation, equity, accountability, and adaptability. Below are five potential models, each with distinct principles, strengths, weaknesses, and feasibility.
1. Decentralised Network Governance
- Description: A distributed model where AI systems are governed through decentralised networks, leveraging blockchain or similar technologies to ensure transparency and collective decision-making. Citizens, organisations, and AI entities collaborate via digital platforms to set rules, monitor compliance and allocate resources.
- Principles: Transparency, participation, adaptability.
- Strengths: Resists centralised control, reducing risks of authoritarianism or corporate monopolies; empowers diverse stakeholders; aligns with decentralised AI economies (e.g., Web3, DAOs).
- Weaknesses: Scalability challenges due to slow consensus mechanisms; vulnerability to misinformation or manipulation; requires high digital literacy, potentially excluding marginalised groups.
- Feasibility: Viable in tech-savvy societies with robust digital infrastructure. Examples like Ethereum-based DAOs and IEEE’s AI ethics councils provide early blueprints. Bridging digital divides is a key challenge.
2. Technocratic Hybrid Democracy
- Description: Combines democratic institutions with technocratic expertise, where elected representatives collaborate with AI specialists and algorithms to craft policies. AI provides real-time data analysis and predictive modeling, while human oversight ensures accountability.
- Principles: Expertise, accountability, efficiency.
- Strengths: Balances democratic legitimacy with technical competence; leverages AI for complex issues like climate change or economic inequality; retains human oversight to mitigate unchecked AI autonomy.
- Weaknesses: Risks elitism, as technocrats may dominate; potential for over-reliance on AI, eroding human judgment; susceptible to capture by powerful tech corporations.
- Feasibility: Feasible in nations with strong democratic traditions and AI ecosystems (e.g., EU, Singapore). Early examples include Singapore’s Smart Nation initiative and AI-driven urban planning. Robust checks are needed to prevent technocratic overreach.
3. Global AI Regulatory Framework
- Description: A supranational body, akin to the United Nations or a new AI-specific organisation, sets standards, enforces regulations, and coordinates AI development. National governments retain sovereignty but align with global protocols to manage cross-border impacts.
- Principles: Universality, cooperation, enforcement.
- Strengths: Addresses global challenges like AI-driven cyberattacks or economic disruption; prevents a “race to the bottom” in ethics; fosters trust through consistent rules.
- Weaknesses: Sovereignty concerns may lead to resistance; bureaucratic inertia slows decision-making; enforcement is challenging without universal agreement.
- Feasibility: Partially implemented through UNESCO’s AI Ethics Recommendation (2021) and EU’s AI Act (2024). Geopolitical rivalries (e.g., US-China tech competition) pose significant hurdles.
4. AI-Augmented Direct Democracy
- Description: AI empowers citizens to participate directly in governance through digital platforms, aggregating public input, filtering noise, and providing personalised policy simulations for real-time referenda.
- Principles: Empowerment, clarity, responsiveness.
- Strengths: Enhances democratic participation, countering apathy; leverages AI for informed decision-making; adaptable to local and global issues.
- Weaknesses: Risks populism and short-sighted policies; digital divides exclude non-tech-savvy populations; susceptible to algorithmic bias or manipulation.
- Feasibility: Early experiments, like Taiwan’s Taiwan platform, show promise. Scalable in connected societies but requires safeguards against misinformation and bias.
5. Post-Scarcity Governance (AI-Driven Resource Management)
- Description: A radical model for a post-scarcity world, where AI optimises resource production and distribution to meet universal needs, rendering traditional economic and political systems obsolete. Governance focuses on managing AI to ensure equity and sustainability.
- Principles: Equity, sustainability, minimalism.
- Strengths: Addresses inequality and scarcity; frees humans from repetitive labor; reduces resource-based conflict.
- Weaknesses: Relies on flawless AI and universal cooperation; risks loss of human agency; transitioning from existing systems is destabilising.
- Feasibility: A long-term vision, with early steps in AI-driven healthcare and energy (e.g., smart grids). Requires cultural and political shifts to embrace post-scarcity values.
AI Ethics Frameworks
Ethical frameworks are essential to guide AI governance, ensuring fairness, transparency, and human-centricity. Below are key frameworks, their principles, applications, and challenges.
1. UNESCO Recommendation on the Ethics of Artificial Intelligence (2021)
- Principles: Human rights, transparency, fairness, sustainability, human oversight.
- Applications: Guides national AI policies (e.g., EU’s AI Act), shapes corporate charters, and informs international standards for AI in healthcare and education.
- Strengths: Broad global consensus enhances legitimacy; addresses cross-border issues like surveillance.
- Weaknesses: Non-binding nature limits enforcement; vague principles lead to inconsistent implementation.
2. IEEE Ethically Aligned Design (2019)
- Principles: Human well-being, accountability, transparency, privacy, bias mitigation.
- Applications: Informs technical standards for robotics, healthcare, and transportation; used in AI ethics training and corporate governance (e.g., IBM).
- Strengths: Practical and inclusive, with global stakeholder input.
- Weaknesses: Technical focus may overlook societal impacts; adoption varies across industries.
3. European Union’s Ethics Guidelines for Trustworthy AI (2019)
- Principles: Human agency, robustness, privacy, transparency, fairness, societal well-being, accountability.
- Applications: Shapes EU’s AI Act, guides public sector AI use (e.g., smart cities), and influences corporate policies in Europe.
- Strengths: Legally enforceable; comprehensive scope.
- Weaknesses: Eurocentric perspective; high compliance costs for smaller organisations.
4. Asilomar AI Principles (2017)
- Principles: Safety, value alignment, human control, responsibility, long-term safety.
- Applications: Guides AI safety research (e.g., OpenAI) and public discourse on risks like autonomous weapons.
- Strengths: Forward-looking, with broad AI community endorsement.
- Weaknesses: Lacks enforcement; some principles are too abstract for immediate use.
5. Corporate AI Ethics Principles (e.g., Google, Microsoft)
- Principles: Fairness, accountability, privacy, beneficence.
- Applications: Shapes AI product design (e.g., Google’s bias audits), corporate governance, and public relations.
- Strengths: Direct influence on AI development at scale.
- Weaknesses: Risks “ethics washing”; lacks external accountability.
Common Themes and Applications
AI ethics frameworks converge on fairness, transparency, accountability, privacy, human-centricity, and global cooperation. They inform governance by:
- Shaping regulations (e.g., EU’s risk-based AI Act).
- Guiding corporate ethics boards and audits.
- Influencing national AI strategies (e.g., Canada’s Directive on Automated Decision-Making).
- Empowering civil society to hold entities accountable (e.g., AlgorithmWatch).
Challenges in AI Governance and Ethics
Implementing AI governance and ethics faces several hurdles:
- Vagueness: Broad principles like “fairness” lack clear metrics, leading to inconsistent application.
- Cultural Relativism: Ethical priorities vary globally (e.g., privacy in EU vs. China), complicating consensus.
- Enforcement Gaps: Voluntary frameworks rely on goodwill, lacking legal or technical enforcement.
- Corporate Influence: Tech giants may prioritise profit over public good, undermining ethical goals.
- Technological Complexity: Rapid AI advancements (e.g., generative AI) outpace guidelines.
- Resource Disparities: Smaller organisations and developing nations lack implementation capacity.
- Existential Risks: Frameworks focus on immediate issues (e.g., bias) while neglecting long-term threats like superintelligence.
Future Directions
To align governance with AI’s potential, future efforts should:
- Standardise Metrics: Develop measurable criteria for fairness and accountability (e.g., bias benchmarks).
- Incorporate Diverse Voices: Include marginalised communities and non-Western perspectives.
- Strengthen Enforcement: Integrate frameworks into binding regulations and treaties.
- Adapt to Emerging Technologies: Update guidelines for new paradigms like neuromorphic computing.
- Promote Capacity Building: Support developing nations through funding and training.
- Balance Risks: Address immediate harms and long-term existential threats.
Conclusion
Human civilisation is an ever-evolving experiment, and no political system, including democratic capitalism, can claim finality. The AI revolution demands new governance models – decentralised networks, technocratic democracies, global frameworks, direct democracies, or post-scarcity systems – to address its economic, social, and political impacts. AI ethics frameworks, from UNESCO to corporate principles, provide normative foundations but must overcome vagueness, cultural divides, and enforcement gaps. Conservative resistance to change risks stagnation; instead, societies must experiment with adaptive, inclusive systems that prioritise human values. As AI reshapes civilisation, the interplay of governance and ethics will determine whether it fosters equity and flourishing or exacerbates inequality and control. The path forward lies in embracing uncertainty, iterating on historical lessons, and building systems that harness AI’s potential for the collective good.
References
- Acemoglu, D., & Robinson, J. A. (2012). Why Nations Fail. Crown Business.
- Bostrom, N. (2014). Superintelligence. Oxford University Press.
- Brynjolfsson, E., & McAfee, A. (2014). The Second Machine Age. W.W. Norton.
- European Commission. (2019). Ethics Guidelines for Trustworthy AI.
- Future of Life Institute. (2017). Asilomar AI Principles.
- Harari, Y. N. (2018). 21 Lessons for the 21st Century. Spiegel & Grau.
- IEEE Global Initiative. (2019). Ethically Aligned Design.
- Jobin, A., et al. (2019). The global landscape of AI ethics guidelines. Nature Machine Intelligence, 1(9).
- UNESCO. (2021). Recommendation on the Ethics of Artificial Intelligence.
- Zuboff, S. (2019). The Age of Surveillance Capitalism. PublicAffairs.